Did two pulses of evolution supercharge human cognition?
An intriguing new study tries to tabulate the ages of genetic variants associated with human phenotypes, but its claims about recent brain evolution may not pan out.
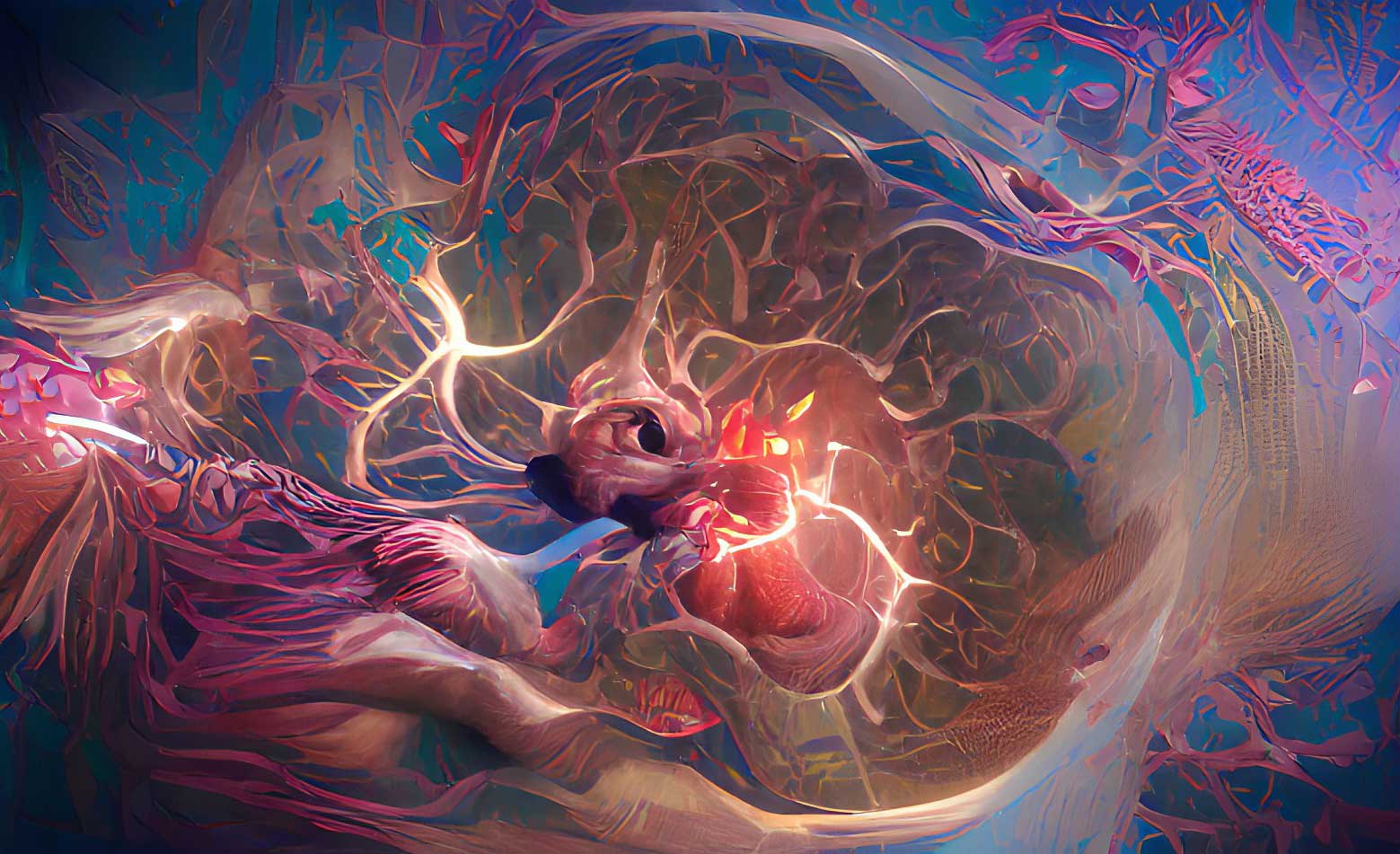
Genetic variation in humans has a timeline, and today geneticists can often estimate the time when a genetic variant first arose in the ancestors of today's people. In principle this timeline can help us understand how human traits evolved, even behaviors like language or cognition that may leave little fossil trace. But there's a big limitation on this approach. We don't know how—or whether—most genetic changes make a difference to phenotypes.
That challenge hasn't stopped scientists from trying to work out an evolutionary timeline for cognitive evolution. In recent years genome-wide association studies have highlighted thousands of gene-phenotype correlations to work with. A new preprint relies on these data to suggest that human brain evolution happened in two pulses—one more than a million years ago, and the other very recently with a peak only around 55,000 years ago.
A reader wrote to ask what I thought about this research so I gave it a close look. The new preprint is the work of Ilan Libedinsky and coworkers, who took the clever approach of combining two sets of data: a catalog of genetic variants associated with phenotypes by genome-wide association studies, and a catalog of estimated ages of genetic variants first published by Patrick Albers and Gil McVean. What Libedinsky and collaborators found looks interesting. Genetic variants associated with phenotypes in today's humans have a Loch Ness monster-shaped distribution: the head and neck corresponding to a recent peak within the last 150,000 years, and an older “hump” with a maximum around 1.1 million years.
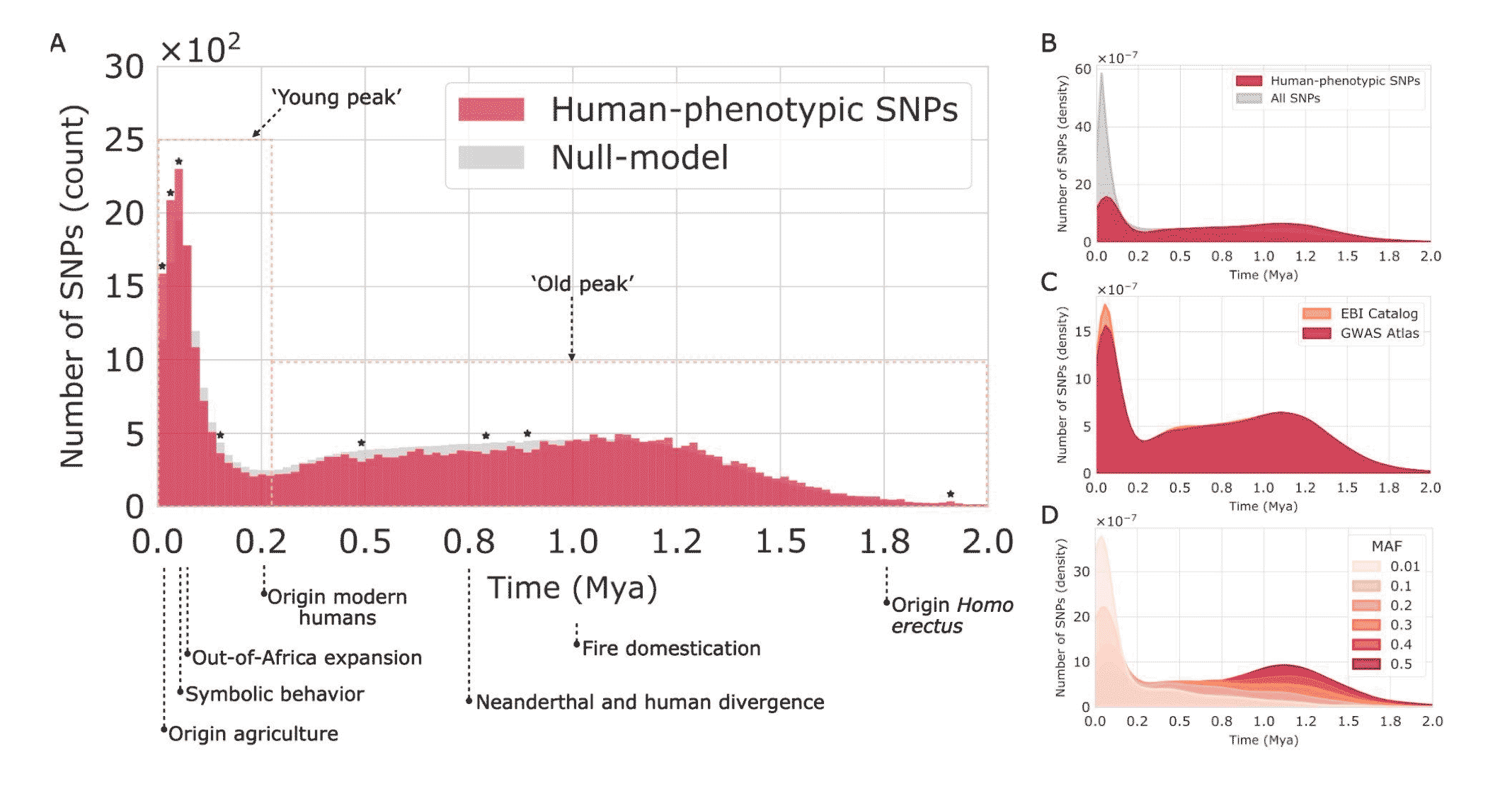
In addition to this analysis, Libedinsky and coworkers looked at different sets of gene-phenotype relationships grouped by functional categories, showing that some have a different pattern of SNP ages. For example, they found that genetic variants associated with “opthalmological traits” have a significantly lower average age than the overall distribution. This series of comparisons gave rise to the title of their paper, emphasizing that the “Genetic timeline of human brain and cognitive traits” is young.
“Gene-set analysis provided evidence that genes containing recent modifications are statistically enriched for genes associated with brain and cognitive phenotypes.”—Ilan Libedinsky and coworkers
Many people who have been reacting to this preprint seem to be misreading what the research can show. I've seen comments suggesting that this is some kind of “supercharged recent evolution” or other evidence of uniquely rapid change in human brain biology. The paper itself ends on a more cautious note, emphasizing that the gene-phenotype associations in today's populations may not have been the same in ancient human ancestors.
For me, I think the biggest “headline” of the paper is actually very trivial. A recent peak in this distribution of genetic variant ages is not in the least bit surprising. It's exactly what we should see if genes were doing nothing at all to phenotypes. But I do think there is much of interest in this approach. Instead of recent evolution, the most interesting message for me is the continuing importance of variation from our ancestors who lived more than a million years ago.
A little population genetics
An early step in any analysis of the timeline of genetic change is to understand what the pattern of random or stochastic change should look like. Most population genetics analyses start with a very simple set of assumptions: random mating, no changes in population size, no natural selection. Humans are more complicated than this, because our ancestors underwent a series of population expansions and episodes of mixture.
This study looked at single nucleotide polymorphisms (SNPs), which are among the best understood variations in human genomes. Nearly all of them result from a single mutation in the germline of a single ancestor; what makes them polymorphic today is that both the new and ancestral variants have survived in one or more populations. The persistence of a variant in any generation depends on the number of offspring of every individual who carries it; it's very likely that a rare variant will quickly become extinct, but there is a small chance it will become common. What this means is that if we look at all the variants in a population and ask how old they are, most of them will be young and fewer will be very old. If the variants are chosen truly randomly from a single sample, their ages will look like an exponential decay curve.
The exact numbers of young and old variants depend on the size of the population and details of its history. Human SNPs in gene-phenotype studies tend to skew older than a randomly selected set, because geneticists don't tend to include extremely rare SNPs in their microarrays. Both this bias and human population history are responsible for the SNPs forming a recent “peak” instead of a decay curve. That peak is visible in the gray color in this figure panel from Libedinsky and colleagues' paper:
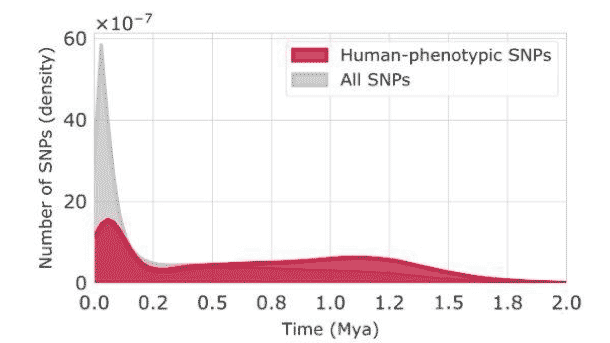
As you can see, a very large proportion of “all SNPs” have estimated ages within the last 150,000 years, with a very high peak in the recent past. These age estimates come from a method developed by Albers and McVean, which relies on the pattern of linkage between each SNP variant and its nearby neighbors.
The most obvious element of the figure above is that the “human phenotypic SNPs” have a much lower peak than “all SNPs”, and many more of them are older than 0.8 million years. Why is that? In this case, there are two reasons and it's not clear which is more important. These SNPs are drawn from genome-wide association studies (commonly abbreviated as GWAS), in which geneticists have compared phenotypes with SNP genotypes in tens or hundreds of thousands of people. Such studies can identify genetic variants that are associated with a very small effect on the phenotype, sometimes highlighting dozens—and occasionally hundreds—of SNPs that are statistically associated with a phenotype in a small way. But the statistical power of this approach depends both on effect size and SNP frequency. As a result, the SNPs with significant phenotype associations are biased toward higher frequencies, which tend to be older. Another panel shows just how much the variant frequency matters: in this chart the MAF is the minor allele frequency, the frequency of the less common of the two variants for each SNP.
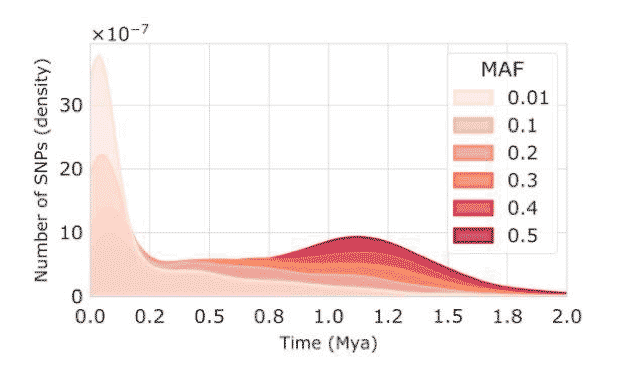
The 1% bin in particular is highly underrepresented because genome-wide association studies have low power to detect phenotype associations for such rare variants.
The other reason why the significant gene-phenotype SNPs are older is purifying selection. When genetic variants do something to the phenotype, they often do something that makes survival or reproduction less likely. Many genome-wide association studies are investigations of diseases or health risks. Rare variants that hurt long-term health are a major focus of human genetic research. Variants that have deleterious effects on survival or reproduction are unlikely to persist long in the population.
So the results show a deficit of young gene-phenotype significant SNPs, and this is totally unsurprising. It's exactly the pattern we should expect genome-wide association studies to produce.
Differences among phenotype categories
This raises a very interesting question about these young SNPs. Libedinsky found that the distribution of ages is different for different kinds of phenotypes. The biggest outlier category is ophthalmological traits, which includes over 900 SNPs with a median age of only around 30,000 years—way younger than the next youngest category, “psychiatric” traits, with some 4000 SNPs averaging more than 400,000 years. What's the story here?
First, remember that even these lowest categories have a deficit of young, low-frequency SNP variants. GWAS and evolution both filter against them. But some GWAS analyses are stacked to identify rare SNPs that may be deleterious due to their association with health problems. Geneticists and clinicians build large cohorts of tens of thousands of people who suffer from certain diseases, comparing them with unaffected people, to find SNPs that are rare in the general population but statistically overrepresented in a disease cohort.
There's a long history now of GWAS for opthalmological traits targeting disorders like macular degeneration and glaucoma. This is also generally true of studies of psychiatric phenotypes such as schizophrenia, bipolar disorder, and depression. It's probable that these have younger average SNP ages because the deck is stacked more toward rare SNP discovery.
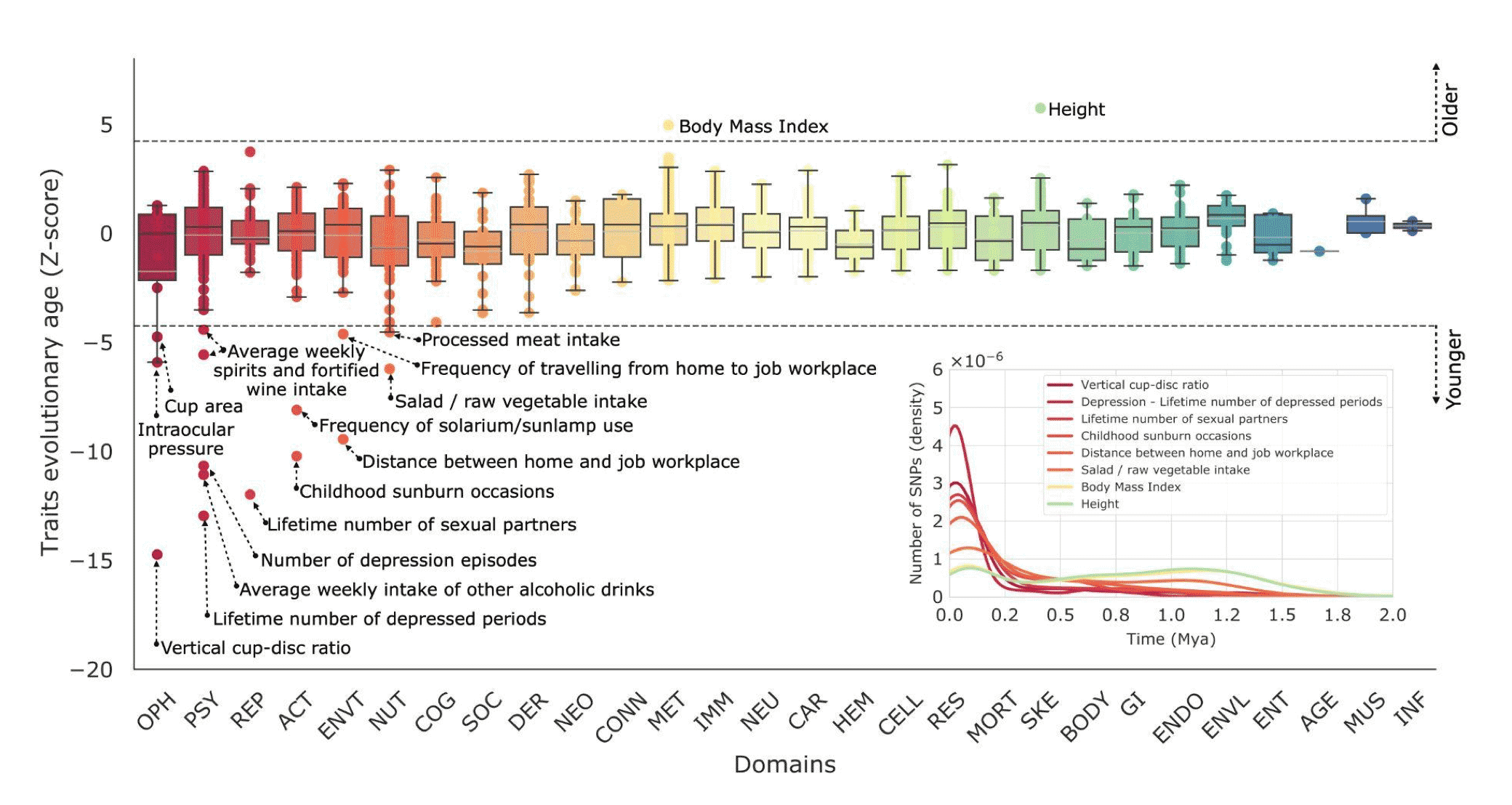
By contrast, Libedinsky found higher median ages for traits labeled as “immunological”, “skeletal”, and “metabolic”, all with median ages above 740,000 years. These kinds of traits may account for some of the Loch Ness monster's hump: ancient SNP variants that have persisted within human ancestral populations for hundreds of thousands of years, still influencing today's phenotypic variation.
When I look at those categories, one thing I notice immediately is that these are exactly the categories that have been most influenced by introgression from archaic human populations. Many humans today have genetic variants from Neandertals or Denisovans that are associated with immune phenotypes. My guess is that this signal may have a real evolutionary explanation.

Still, the first thing to rule out is some kind of discovery bias toward older SNPs in these categories. That will require more drilling into the details of GWAS for these categories than I can do right now.
I will point out that the trait in the “skeletal” phenotype with the oldest median SNP age is stature. Geneticists have examined stature in more large GWAS studies than any other trait, resulting in literally thousands of associated SNPs, most with miniscule effect sizes. What's evident in the lower right panel of the figure above, is that stature—here labeled “height”—together with body mass index, both show a distinctive Loch Ness monster hump. This seems curious to me. It's strange that stature should stand out as uncharacteristic in having so few young SNPs. I think more investigation of the source data are needed to understand this.
More on the old SNPs
Introgression may be a great explanation for why old variants may be overrepresented among GWAS-significant SNPs. But there are two other explanations worth examination: Either an earlier large population size followed by bottleneck, or balancing selection.
All three of those explanations can give rise to a similar pattern of genetic variation, depending on what is measured. For example, back in 2010 Chad Huff, Alan Rogers, and coworkers arrived at an interesting picture of human population size. They did not have whole genomes to work with, as we do today, so they used a clever method to look at genetic variation around a category of mobile genetic elements known as Alu insertions. They showed that the human population seemed to have a larger size more than a million years ago, followed by a reduction in size. More recent work has shown a broadly similar picture. The ancestral population of humans, Neandertals, and Denisovans seems to have harbored more genetic diversity than any of those branches did later, including the African branch that makes up most of the Middle Pleistocene ancestry of humans worldwide. Rogers together with Henry Harpending proposed this kind of “long-necked bottle” scenario back in the 1990s, and it remains a viable concept.
Introgression may contribute to that pattern also. I showed in 2017 that SNP variation from ancestral population structure could mimic the appearance of an ancestral large population, and a lot of recent attention has gone to understanding possible ghost introgression from ancient African groups. Ultimately it’s an empirical question; ancient DNA can show whether the SNPs are in Neandertals or Denisovans, and some analysis of recent genomes can show if they are clustered together on haplotypes that look introgressed.
Balancing selection also can yield a similar pattern, but localized within parts of the genome that are associated with phenotypes under selection. Since this new work is focused on phenotypes, balancing selection is worth a look to explain old SNP distributions.
In March, Alber Aqil and coworkers had a new paper in eLife that surprised me. They looked at deletion polymorphisms in human populations today—that is, regions of the genome where a person may carry both a long and short allele, which differ in length by more than 50 base pairs. They found that many of these deletion polymorphisms are very old in humans, dating back hundreds of thousands of years. A very high fraction of them are shared as polymorphisms not only in today's populations but also in Neandertals. Compared to recent polymorphisms, these ancient deletion polymorphisms were more likely to include GWAS associations and to intersect with coding regions of genes. This suggests that there has been a lot of balancing selection maintaining these polymorphisms in both African and Neandertal human ancestors, continued from the population of their common ancestors more than 700,000 years ago.
“Our results suggest that balancing selection resulted in ancient deletions harboring disproportionately more exonic variants with GWAS (genome-wide association studies) associations.”—Alber Aqil and coworkers
Aqil and coworkers found a broadly similar pattern for SNPs. I would say that Libedinsky and coworkers have partially replicated this result, finding ancient GWAS SNPs. That puts balancing selection into the mix as an explanation for why some traits may have a very old SNP age distribution. With traits like stature, which might have been under stabilizing selection in many populations of the past, it seems possible that long-term balancing selection on some SNP variants (or other genetic variants) may be part of the picture.
What about the brain?
The last thing of interest in the Libedinsky preprint is the examination of SNPs associated with phenotypes that were assessed from neuroimaging in part of the UK Biobank sample. On the surface, this looks like the neatest result in the paper:
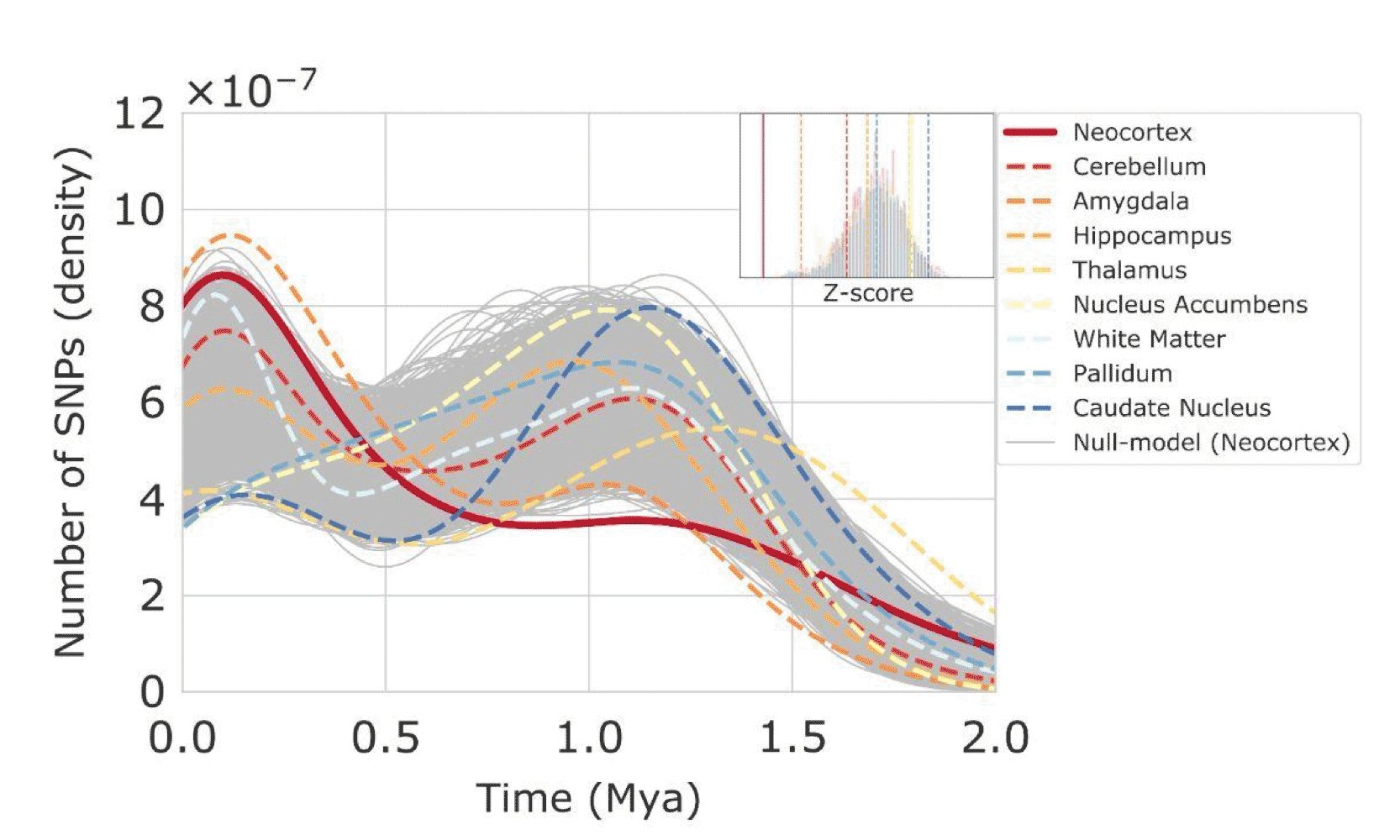
The brain areas seem to have really different SNP age distributions. It would appear that caudate nucleus shows the same large-humped pattern as stature and BMI, while neocortex seems to have a much more recent peak and lower number of old SNP variants. These results seem much more salient than the lumped GWAS SNPs from earlier studies, because these were acquired on the same sample using the same approach. But the number of SNPs in each category is much smaller: Libedinsky and coworkers state that 130 SNPs are included for neocortex. The highly-smoothed lines in the figure are compiled from noisy data, and the multitude of thin gray lines, based on resampling across all SNPs, show how bad the noise really is. Only the neocortex pattern is significantly different from the others, and not by much. Since the neocortex is the biggest part of the human brain, I tend to think that the explanation here is simply that variation in neocortex may be catching more SNPs under purifying selection.
Libedinsky and coworkers included one other kind of analysis that they suggest makes brain and cognitive evolution stand out from other traits. This one centered on coding regions of genes instead of GWAS SNPs, and the authors computed a median age for each gene based on the age distribution of coding SNPs. They then filtered to the 2000 genes with the youngest median ages, and did a category analysis on these to see if some categories of genes are overrepresented. They also looked at the age distributions for categories of genes without the filtering. Their interpretation of the results is that genes under stronger selective constraint have younger median SNP ages.
“An estimate of the evolutionary age of each gene was derived by computation of the median age of the SNPs located within the start and stop position of each gene, resulting in date estimates for 18,328 human genes.”—Ilan Libedinsky and coworkers
This is a tricky analysis to evaluate, because coding regions of different genes are very different in length, and the age estimates of SNPs within a short span such as a single exon are not independent of each other. It is totally unremarkable that genes under tighter evolutionary constraint should have younger coding SNP distributions. Gene ontology categories have only a loose association with phenotypes; one phenotype may be affected by genes that overlap across categories. What I cannot judge is whether the few differences between gene ontology categories are driven by large differences in a handful of genes. If so, it is probably not very revealing about the timeline of evolution of phenotypes.
Bottom line
It's not a new idea to identify genetic causes of human brain evolution by examining genetic timelines. More than twenty years ago, a group led by Ajit Varki noted that the sialic acid N-glycolylneuraminic acid (Neu5Gc) had been knocked out in human ancestors by the deactivation of the gene CMAH, estimated around 2.2 million years ago. This change came before some hominin lineages evolved larger brains, which seemed significant due to the differential regulation of Neu5Gc in the brains of chimpanzees and other primates. It seemed possible that the loss of this sialic acid released neural tissue from some longstanding constraint.
Similar work of this kind followed. Some research teams focused on other gene losses or duplications, such as the loss of the heavy-chain myosin encoded by MYH16 some 2.5 million years ago, or the partial duplication of the ARHGAP11A gene resulting in human ARHGAP11B, involved in Rho-signaling during neurogenesis and shared with Neandertal and Denisovan genomes. For more than fifteen years, James Sikela has led a team carrying out work on a protein domain known as DUF1220. With more than 250 copies in human genomes, and fewer than 130 in other apes, this is the most amplified functional protein domain in our evolution, and copy number variation affects several neurological conditions in living people.
Meanwhile, other teams trawled through the genome looking for evidence of positive selection on genes related to neural development. The work by Bruce Lahn's group on MCPH1 and ASPM was of this kind, both genes showing evidence of recurrent amino acid changes in human ancestors compared to other great apes. A research group led by Gregory Wray focused on promoter regions of genes, finding in 2007 that genes associated with neural processes had been more often affected by positive selection on their promotor regions than other kinds of genes. And there are others, like the fascinating human-accelerated regions (HARs) first studied by Katharine Pollard, the importance of which remains uncertain.
Not all of these have continued as substantial directions of research, and some have been false starts. As we try to build knowledge of how human brains and cognition evolved, we will need more integrative research that pulls together many genetic signals. This new preprint by Libendinsky and coworkers is an intriguing step toward such integrative research.
Still, whatever we find about brains and behavior in recent humans has to fit with the broader evolutionary record of hominins. We know from comparison with Neandertal and Denisovan genomes that very few genetic changes are both unique and fixed in living people. Complex phenotypes are by definition influenced by many genes.
I will emphasize one other important limitation of this work. Although more than 60,000 GWAS SNPs are a very large number, the GWAS approach has a very strong population bias because geneticists have allocated much greater effort to examining European ancestry populations. This chart from Giorgio Sinugo and coworkers shows the extent of overrepresentation of European ancestry in 2019:
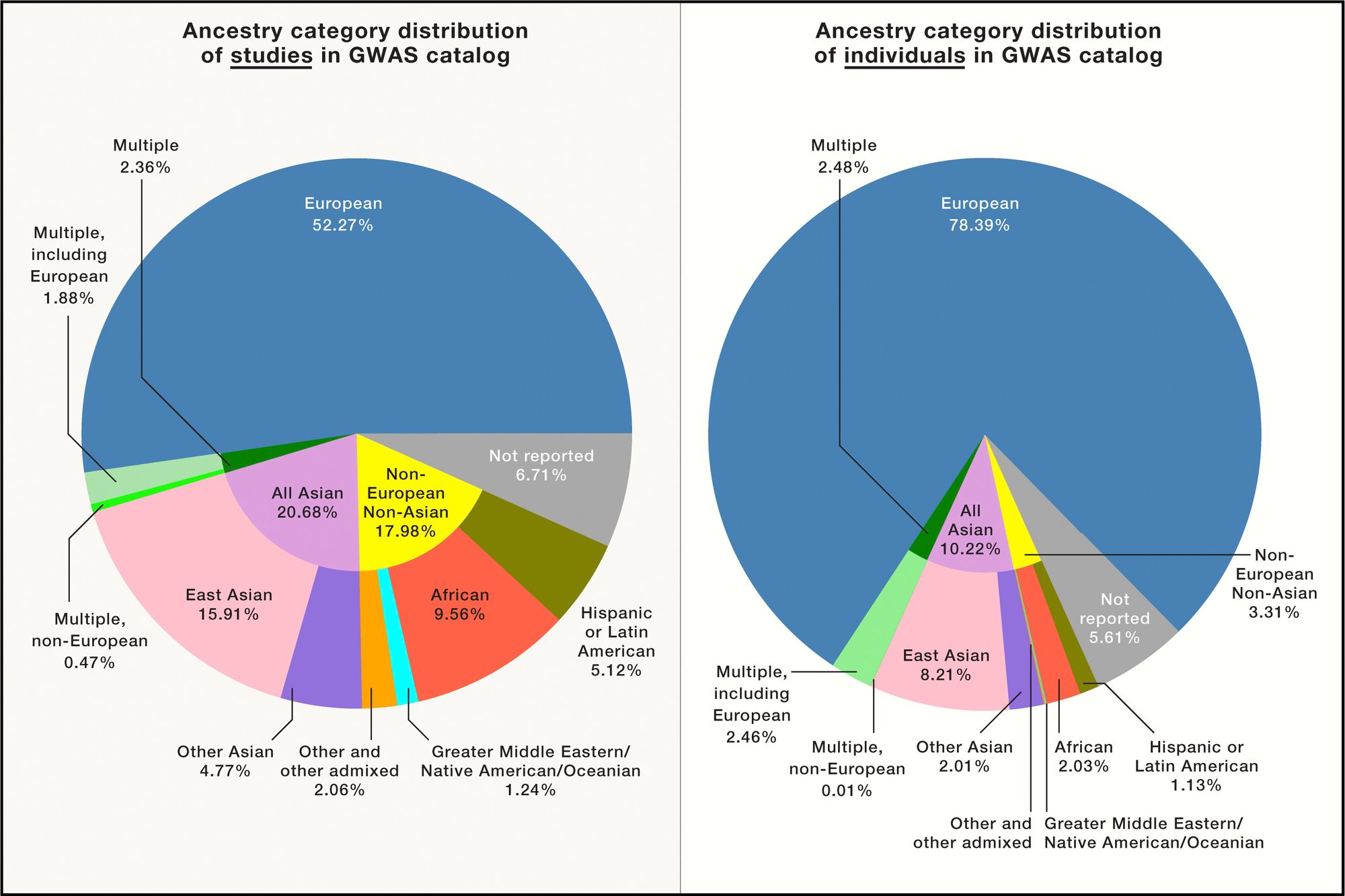
Almost 80% of the individuals from which GWAS SNPs are discovered—where the number of participants is the main constraint on statistical power—are of European ancestry. The present study by Libedinsky and coworkers includes more than 60,000 GWAS SNPs, which is a large number, but these do not represent gene-phenotype relationships in most human populations. Their age distributions in other populations may be the same, but we don't know yet.
So to answer the question in my post headline, we don't know if any parts of human brain or behavioral evolution happened in pulses. What may look like a recent “peak” of genetic change is in fact a deficit from an expected high number of recent variants. This deficit can be explained by discovery bias and purifying selection, and it doesn't really say anything about the possible direction or magnitude of phenotypic change.
More interesting is the overrepresentation of old SNPs associated with some phenotypes. Some substantial parts of today's human phenotypic variation date back long before our African ancestors began to separate from the ancestors of Neandertals and Denisovans. Some of the variants that matter to phenotypes may have introgressed into recent humans from these archaic ancestors, while others persisted in all these populations. The extent that selection has retained and reshuffled variation from ancestors who lived more than a million years ago is a growing story that is developing on several fronts.
Notes: Lots of references below into some of the primary literature on evolution of genes and brains.
My reader asked how the new idea about recent evolution of brain and behavior might intersect with my own work on recent positive selection in humans. Positive selection has been a distinctive aspect of recent human evolution, with hundreds of long haplotypes in today's humans showing evidence of this process. In many instances, there are good hypotheses about which phenotype was the target of positive selection, while some remain obscure.
There are several important limitations on GWAS that I did not discuss in the post, but that affect the interpretation of the pattern of phenotypic evolution. Most GWAS are carried out on SNP arrays that are sparse relative to the entire genome. The approach relies on the principle that nearby SNPs are physically linked, so that variants that cause phenotypic differences may be detected without being directly genotyped. The fact that GWAS SNPs may be in this sense “innocent bystanders” is itself a source of bias on their age distribution. This becomes more important when the causal SNP has been increasing in frequency under selection; linked SNPs on the same haplotype will generally be older. GWAS SNPs certainly include some SNPs on positively selected haplotypes but these may not be well aged; they are numerically few compared to neutral or slightly deleterious SNPs that affect phenotypes.
The main point is that GWAS imposes pretty extreme discovery biases that confound population genetic analysis of SNP samples. We're not going to be able to say much about how phenotypes evolved based on GWAS SNPs unless these biases are better understood.
References
Albers, P. K., & McVean, G. (2020). Dating genomic variants and shared ancestry in population-scale sequencing data. PLOS Biology, 18(1), e3000586. https://doi.org/10.1371/journal.pbio.3000586
Aqil, A., Speidel, L., Pavlidis, P., & Gokcumen, O. (2023). Balancing selection on genomic deletion polymorphisms in humans. ELife, 12, e79111. https://doi.org/10.7554/eLife.79111
Chou, H.-H., Hayakawa, T., Diaz, S., Krings, M., Indriati, E., Leakey, M., Paabo, S., Satta, Y., Takahata, N., & Varki, A. (2002). Inactivation of CMP-N-acetylneuraminic acid hydroxylase occurred prior to brain expansion during human evolution. Proceedings of the National Academy of Sciences, 99(18), 11736–11741. https://doi.org/10.1073/pnas.182257399
Florio, M., Albert, M., Taverna, E., Namba, T., Brandl, H., Lewitus, E., Haffner, C., Sykes, A., Wong, F. K., Peters, J., Guhr, E., Klemroth, S., Prüfer, K., Kelso, J., Naumann, R., Nüsslein, I., Dahl, A., Lachmann, R., Pääbo, S., & Huttner, W. B. (2015). Human-specific gene ARHGAP11B promotes basal progenitor amplification and neocortex expansion. Science, 347(6229), 1465–1470. https://doi.org/10.1126/science.aaa1975
Hawks, J. (2017). Introgression Makes Waves in Inferred Histories of Effective Population Size. Human Biology, 89(1), 67–80. https://doi.org/10.13110/humanbiology.89.1.04
Haygood, R., Fedrigo, O., Hanson, B., Yokoyama, K.-D., & Wray, G. A. (2007). Promoter regions of many neural- and nutrition-related genes have experienced positive selection during human evolution. Nature Genetics, 39(9), Article 9. https://doi.org/10.1038/ng2104
Herráez, D. L., Bauchet, M., Tang, K., Theunert, C., Pugach, I., Li, J., Nandineni, M. R., Gross, A., Scholz, M., & Stoneking, M. (2009). Genetic Variation and Recent Positive Selection in Worldwide Human Populations: Evidence from Nearly 1 Million SNPs. PLOS ONE, 4(11), e7888. https://doi.org/10.1371/journal.pone.0007888
Huff, C. D., Xing, J., Rogers, A. R., Witherspoon, D., & Jorde, L. B. (2010). Mobile elements reveal small population size in the ancient ancestors of Homo sapiens. Proceedings of the National Academy of Sciences, 107(5), 2147–2152. https://doi.org/10.1073/pnas.0909000107
Libedinsky, I., Wei, Y., Leeuw, C. de, Rilling, J., Posthuma, D., & Heuvel, M. P. van den. (2023). Genetic timeline of human brain and cognitive traits (p. 2023.02.05.525539). bioRxiv. https://doi.org/10.1101/2023.02.05.525539
Mekel-Bobrov, N., Gilbert, S. L., Evans, P. D., Vallender, E. J., Anderson, J. R., Hudson, R. R., Tishkoff, S. A., & Lahn, B. T. (2005). Ongoing Adaptive Evolution of ASPM, a Brain Size Determinant in Homo sapiens. Science, 309(5741), 1720–1722. https://doi.org/10.1126/science.1116815
Stedman, H. H., Kozyak, B. W., Nelson, A., Thesier, D. M., Su, L. T., Low, D. W., Bridges, C. R., Shrager, J. B., Minugh-Purvis, N., & Mitchell, M. A. (2004). Myosin gene mutation correlates with anatomical changes in the human lineage. Nature, 428(6981), Article 6981. https://doi.org/10.1038/nature02358
John Hawks Newsletter
Join the newsletter to receive the latest updates in your inbox.